New Tool Improves Outcomes of Single-Cell Research
Research By: Erica DePasquale | Nathan Salomonis, PhD
Post Date: November 5, 2019 | Publish Date: Nov. 5, 2019
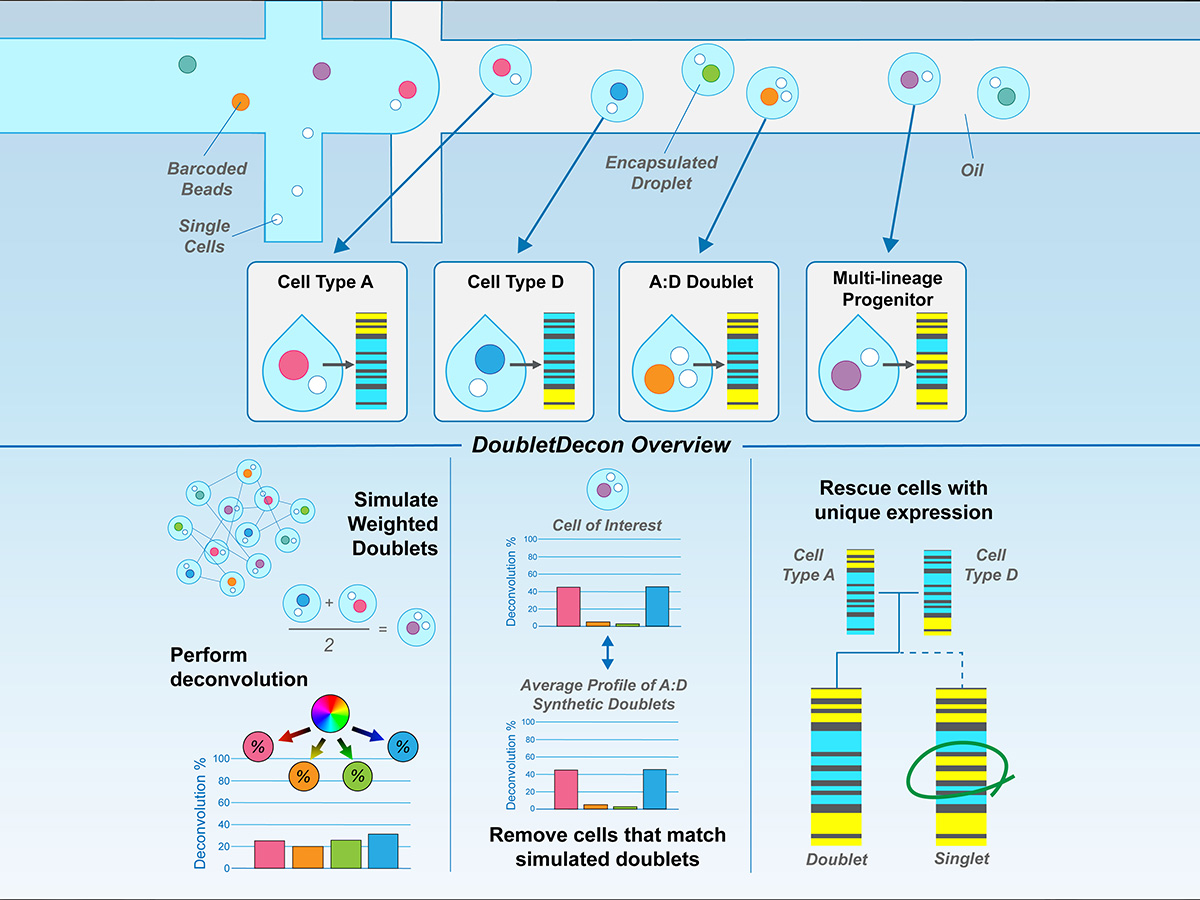
There are trillions of cells in our bodies, and each is unique. An individual cell’s structure and function can be the key to understanding what drives disease. Researchers at Cincinnati Children’s have developed a new computational tool to improve single-cell RNA sequencing datasets, revealing a more complete picture of human cells.
The new tool, DoubletDecon, is introduced in the journal Cell Reports. Researchers in the Salomonis Laboratory in the Division of Biomedical Informatics led the development of DoubletDecon, including first author Erica DePasquale, PhD candidate.
Identifying the nuances of cells
What makes each cell unique? In recent years, single-cell RNA sequencing (scRNA-Seq) has emerged as a means to examine the expression profiles of individual cells. These new technologies have opened the door for studying disease processes in greater detail—instead of studying a tissue as a whole, we can now define cell types and states to understand how they are driving disease.
One of the most popular methods, droplet-based scRNA-Seq, captures single cells in nanoliter-sized water droplets. This allows access to an unprecedented number of cell transcriptomes—but the process is not perfect. As single-cell datasets increase in size, so do potential sources for error.
“Like all new technologies, single-cell RNA sequencing comes with its own set of complications,” says DePasquale. “Droplet-based single-cell sequencing is designed to capture one cell in a single droplet, but sometimes things go awry.”
When two cells are captured, this is called a doublet. These doublets can significantly affect the analysis and interpretation of data. To overcome this complication, DoubletDecon identifies doublets and removes them from datasets, an important new step in single-cell sequencing.
Removing, reclustering, and rescuing cells
First, DoubletDecon generates many simulated doublets by taking weighted averages of two random cells in the dataset. The simulated and original cells are then processed with deconvolution, a mathematical method for understanding the make-up of a sample.
Next, the tool performs a three-step process to:
- Remove cells that look like simulated doublets;
- Recluster those cells to find sets of doublets;
- and Rescue cell sets from incorrect doublet classification.
While doublet detection is a relatively new concept, DoubletDecon is not the only computational approach available. What makes it unique? This tool was designed with a broad range of cell states in mind.
“While we like to think of cells as fitting into neat bins, called ‘cell types,’ biology is much more complicated than that,” says DePasquale. “There are stem cells, cells that transition from early to later stages, and those that flow between multiple cell states depending on the cellular environment. DoubletDecon is the only method that searches for this information in the ‘Rescue’ step to prevent these cells from being incorrectly flagged as doublets.”
Learning how disease impacts cells
Just one cell can tell us a lot about how our bodies work. Single-cell technology allows researchers to study disease mechanisms all the way down to the level of an individual cell. With an ever-increasing volume of datasets, DoubletDecon could have a profound impact on the way single-cell research is performed.
“It’s important that the medical insights derived from these experiments are biologically sound,” says DePasquale. “By providing researchers with a method for removing doublets in datasets with diverse cell states, we are able to correct technical errors and help ensure that the most meaningful conclusions are drawn from the data.
“Doctors and patients rely on good quality research to provide ideas for newer and better treatments. We believe that a solid foundation in laboratory experimentation is the key to their success.”
Access the open-source DoubletDecon tool here.
— This post was written by Dakota Campbell, Division of Biomedical Informatics
Original title: | DoubletDecon: Deconvoluting doublets from single-cell RNA-sequencing data |
Published in: | Cell Reports |
Publish date: | Nov. 5, 2019 |
Research By
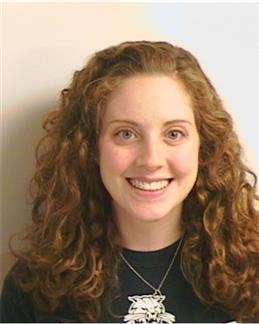
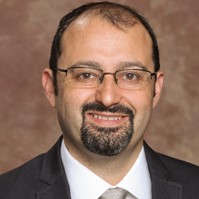