Detecting Abnormal Mental Health Trajectories
Post Date: November 3, 2022 | Publish Date:
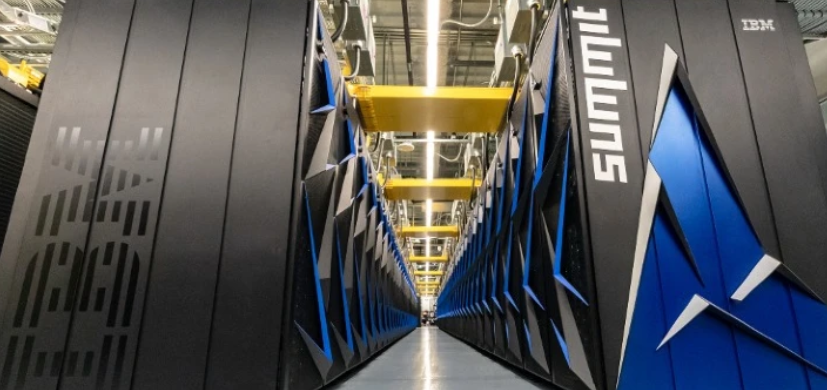
The Mental Health Trajectories program at Cincinnati Children’s is developing tools that combine information routinely collected by doctors with geographic, environmental and other data. Artificial intelligence (AI) then, over time, identifies kids at greatest risk of developing a mental illness for early care and intervention.
Just as pediatric growth charts plot the course of a child’s height and weight over time, these tools aim to do the same for the far more complex trajectories of anxiety, depression and suicide ideation.
The project builds on the work of John Pestian, a neuropsychiatric AI scientist who co-directs the Decoding Mental Health Center at Cincinnati Children’s. For more than 20 years, Pestian has been developing machine-learning methods for identifying individuals at risk of suicide. Using speech samples from therapy sessions and notes from people who died by suicide, Pestian and colleagues showed that AI algorithms could detect suicidality with up to 90% accuracy4.
Achieving the goals of the trajectory project will be no small task. To be accurate, the algorithm needs to be trained on huge data sets. The project is making use of records from 1.3 million patients cared for at Cincinnati Children’s, whose 9 million visits generated both structured and unstructured data. Unstructured data requires natural language processing, a particularly complex technique.
A standard computing cluster would not be up to the job, says Tracy Glauser, associate director of the Cincinnati Children’s Research Foundation and co-director of the Decoding Mental Health Center.
“We realized that the size of the problem would require world-class supercomputing.”