DeepImmuno: a Tool for Creating Custom T-cell Vaccines
Research By: Frank Li | Surya Prasath, PhD | Yizhao Ni, PhD | Nathan Salomonis, PhD
Post Date: May 19, 2021 | Publish Date: May 3, 2021
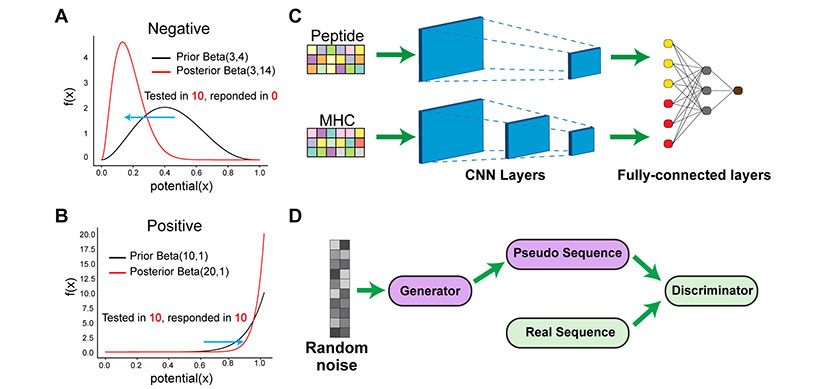
Every year, as many as 18 million new cases of cancer are reported, resulting in more than 9 million deaths throughout the world. One of the most promising recent avenues for treating cancer is by harnessing and reprogramming an individual’s own immune system to find and target specific cancer cells.
Such strategies, referred to as immunotherapies, include cancer vaccines targeting specific cancer peptides (protein fragments exposed on the surface of a cell), uniquely produced by tumor cells. Beyond cancer, similar vaccine strategies may hold promise in other diseases, including infection by deadly viruses such as SARS-Cov-2 (COVID-19).
In both of these examples, predicting which peptide molecules will be recognized by the immune system to elicit an immune response, represent an essential pre-clinical step towards designing and testing new targeted therapies. In a single individual, thousands or millions of potential immunogenic peptides may be produced, with only a few of these representing clinically viable targets. Even more challenging, an immunogenic peptide produced in the cells of one patient may not induce any immune response in another.
To solve this challenge, an interdisciplinary team of researchers from Cincinnati Children’s turned to advanced artificial intelligence (AI) to create and train new models to better predict which peptides will mount an immune response. While in recent years, AI has transformed a number of biomedical disciplines, it has not been extensively evaluated in predicting how to best mount an immune response in life threatening diseases.
Researchers in the laboratories of Nathan Salomonis, PhD, Surya Prasath, PhD, and Yizhao Ni, PhD, tested and evaluated diverse machine, deep learning, and conventional approaches for predicting immunogenicity on real world datasets. They found that a particular class of deep learning methods, called convolutional neural networks or CNN, outperformed existing state-of-the-art methods, improving the prediction of which prior validated cancer or SARS-Co-V-2 peptides will mount an immune response. They call this method DeepImmuno-CNN.
As a potential application of DeepImmuno-CNN, lead author Guangyuan (Frank) Li—a second-year Biomedical Informatics PhD student—chose to test emerging variants of SARS-CoV-2, that have recently proliferated in South Africa, South America, and the UK.
Newly approved RNA-based vaccines for COVID-10 act by triggering B-cells to produce antibodies against specific SARS-CoV-2 spike protein sequences. However, these vaccines are less effective against newly emerging SARS-CoV-2 variants.
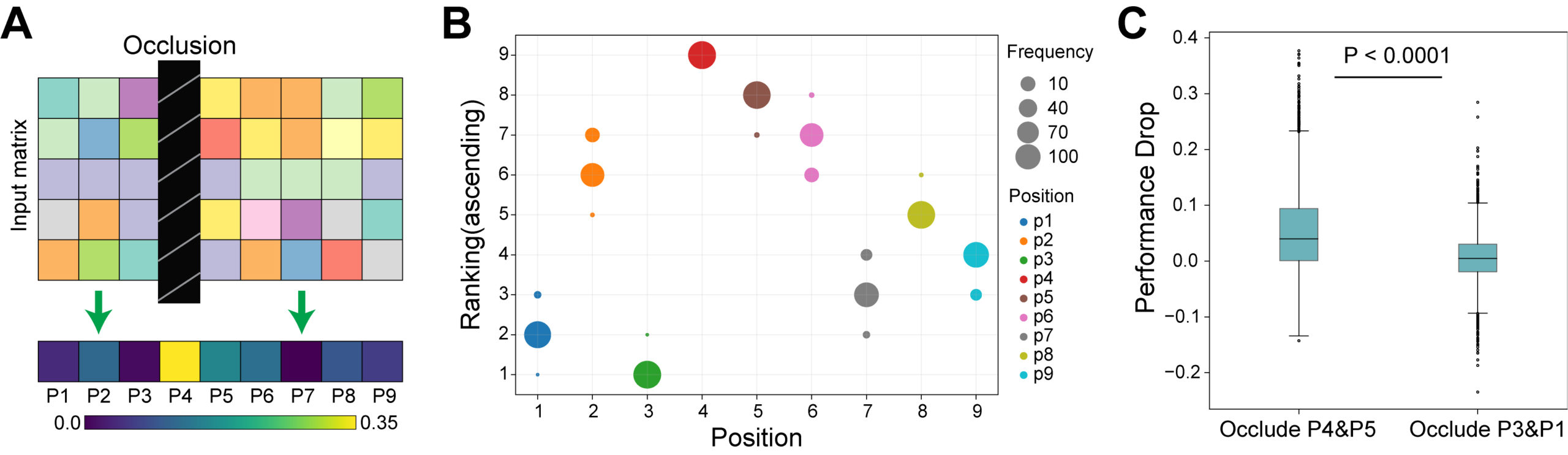
When applied to three of the most common variants (D614G, E484K, N501Y), DeepImmuno-CNN found that the N501Y mutation, frequently found in South Africa and UK variants, is predicted to uniformly increase T cell reactivity across 10 common human HLA alleles, making it a strong candidate for potential novel T cell-based therapies in diverse genetic backgrounds.
Beyond predicting immunogenicity, the study team went a step further to simulate new peptides that don’t naturally exist in nature and are likely to induce an immune response. The utility of such an approach is broad, including the creation of inducible “kill” switches in cells, to target them for destruction, as well as the engineering of synthetic peptides to escape immune responses.
Applying a computational strategy applied in image analysis, (Generative Adversarial Network or GAN), the authors were able to produce a new algorithm, DeepImmuno-GAN, that was able to learn the biochemical interactions that underlie immune responses to produce new synthetic immunogenic peptides.
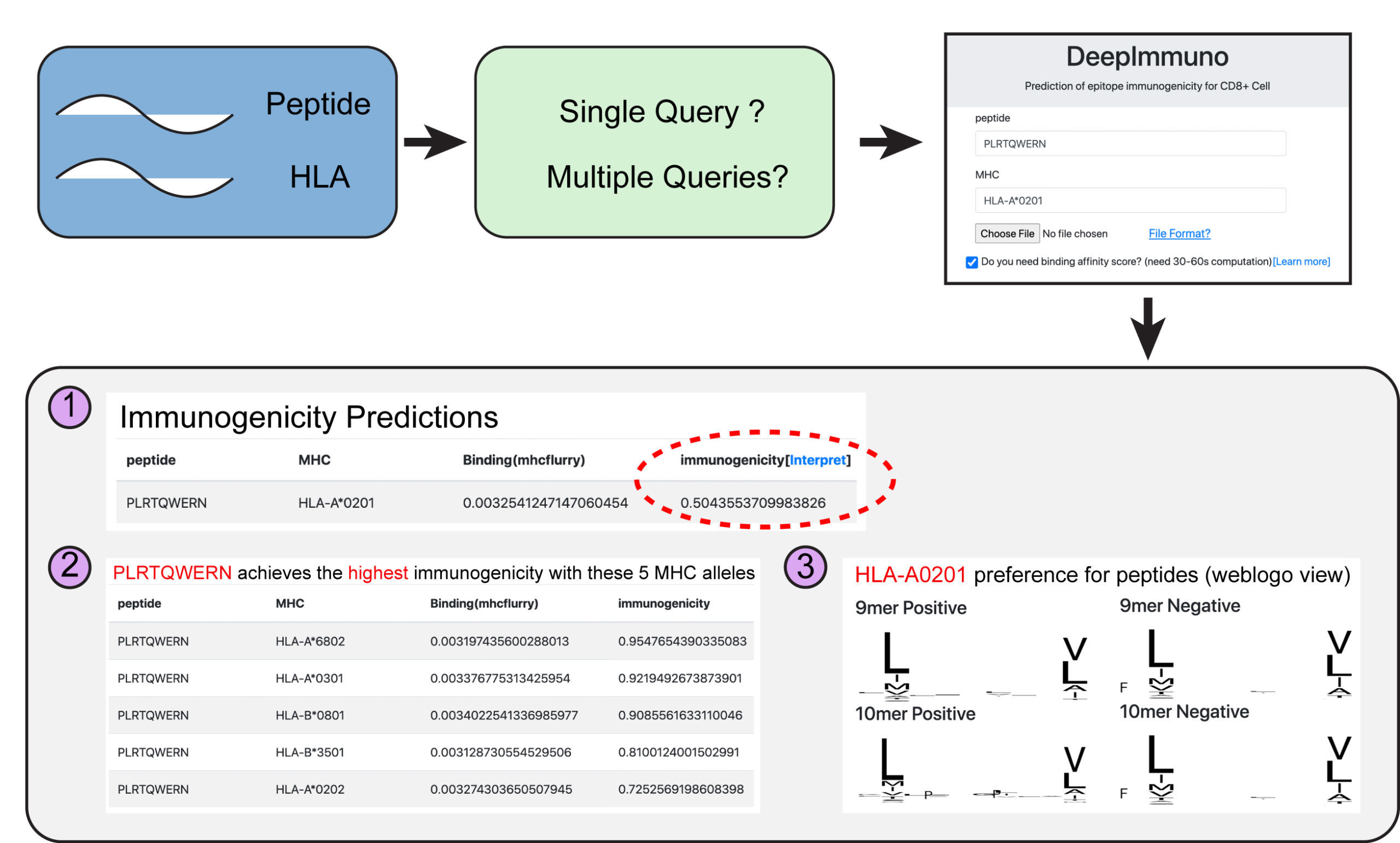
To enable broad re-use of DeepImmuno-CNN, the authors created a free web portal called DeepImmuno for broad re-use by the genomics community. In the future, the authors anticipate these computational strategies will lead to fast, customized solutions for the creation of T-cell vaccines, targeted to an individual’s own genome.
Original title: | Skip Nav Destination Article Navigation DeepImmuno: deep learning-empowered prediction and generation of immunogenic peptides for T-cell immunity |
Published in: | Briefings in Bioinformatics |
Publish date: | May 3, 2021 |
Research By
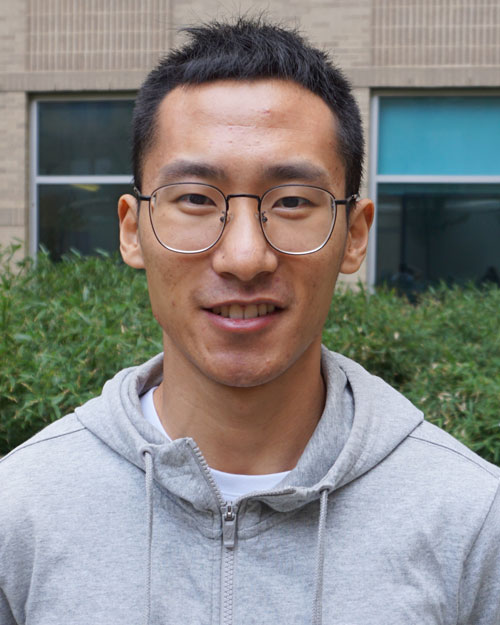
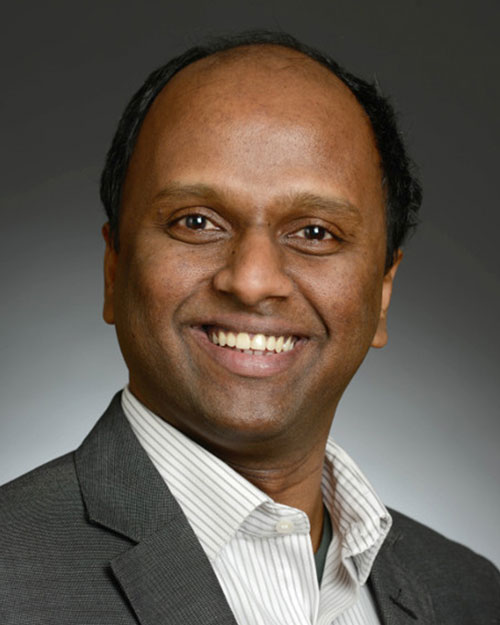
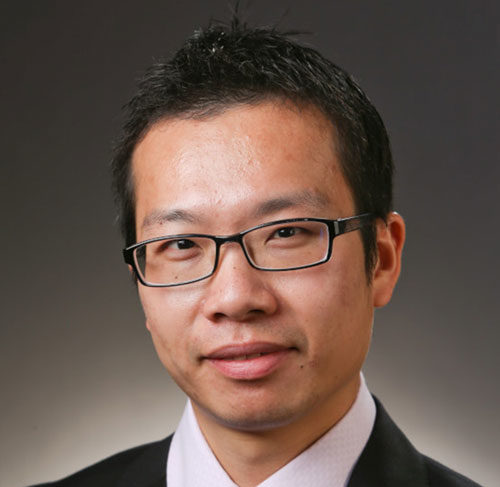
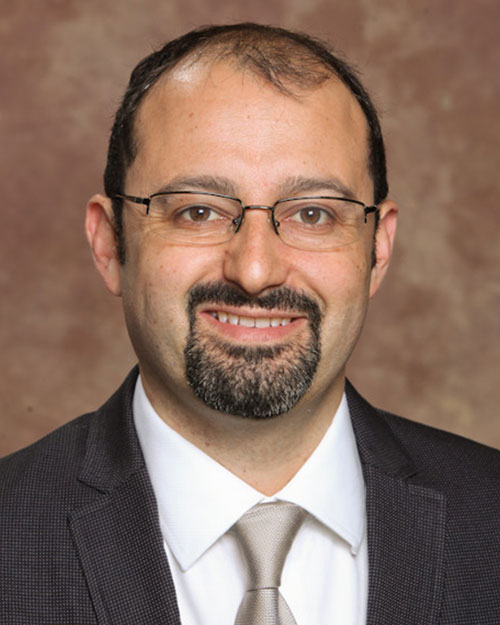