Artificial Intelligence Holds the Key to Diagnosing Rare Esophageal Food Allergy Disease
Research By: Marc Rothenberg, MD, PhD | Margaret Collins, MD
Post Date: October 25, 2021 | Publish Date: June 16, 2021
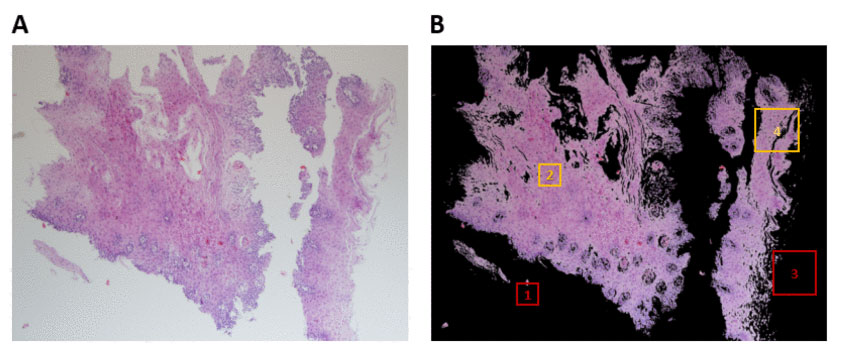
“This research demonstrates that a computer can be trained to diagnose EoE. Actually, it appears that it may do a better job than humans.”
–Marc Rothenberg, MD, PhD
Machine learning is improving diagnosis of eosinophilic esophagitis (EoE), a chronic, allergic inflammatory disease. Experts at Cincinnati Children’s and the Technion–Israel Institute of Technology explain how artificial intelligence (AI) can be used to diagnose and analyze EoE in a study published in the IEEE Open Journal of Engineering in Medicine and Biology.
EoE occurs when a type of white blood cell, the eosinophil, accumulates in the esophagus. This elevated number of eosinophils can release substances into surrounding tissues that cause injury and inflammation to the esophagus. Damaged esophageal tissue can lead to difficulty swallowing or cause food to get stuck when you swallow, potentially resulting in poor growth and chronic pain.
Since EoE can mimic other more common conditions such as gastroesophageal reflux disease, upper endoscopies with biopsies are required to make the diagnosis. A pathologist then reviews those biopsies to determine whether eosinophils are present. However, due to the small size and patchy presence of the features that help distinguish EoE from other diseases, it’s often difficult to standardize a diagnosis from these samples.
In this study, the research team developed an AI-based platform that can more easily classify esophageal biopsies. Using computer vision, the platform analyzes biopsy imagery to identify changes in tissue structure that may not be seen under a microscope by the naked eye.
“This represents a transformative leap forward,” says co-author Marc Rothenberg, MD, PhD, of the Division of Allergy and Immunology. “This research demonstrates that a computer can be trained to diagnose EoE. Actually, it appears that it may do a better job than humans.”
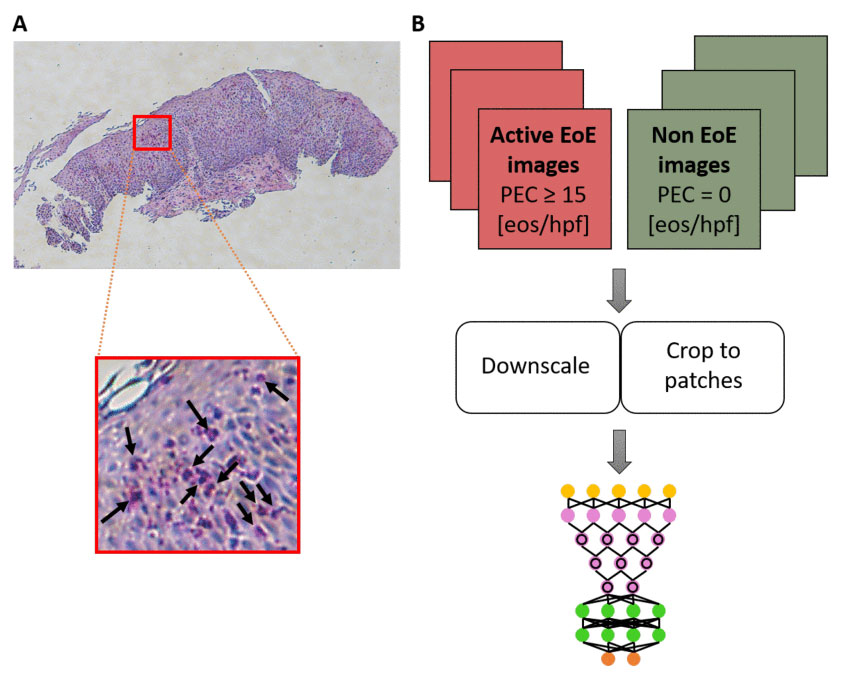
Among samples from patients with EoE and control subjects, researchers found that the platform classified esophageal biopsies with an accuracy of 85 percent, sensitivity of 82.5 percent, and specificity of 87 percent. This automated process—which can be applied to other conditions that rely on biopsy diagnostics—removes human error, ultimately speeding up time to diagnosis.
“We have harnessed deep learning not only to classify patients according to their condition, but also to infer hidden information suggesting that EoE manifests in local features as well as global tissue changes,” says co-author Yonatan Savir, PhD, of the Technion–Israel Institute of Technology.
The idea for this multidisciplinary approach emerged when Rothenberg and others in the Cincinnati Center for Eosinophilic Disorders reached out to computer scientists at the Technion–Israel Institute of Technology, a technology and engineering institution based in Haifa, Israel that has its own medical faculty and affiliated hospital.
Just recently, Cincinnati Children’s launched a new data-driven research program in collaboration with the Technion, called the “Bridge to Next-Gen Medicine,” which is expected to include joint workshops, online lectures, faculty/student exchange visits, and research projects. The two institution’s hope to elevate pediatric medicine on a global scale by leveraging Cincinnati Children’s expertise in patient care, basic research, and translational research with Technion’s excellence in computer science, engineering, and bioinformatics.
“It provides hope for patients to know that scientists within different disciplines are working together to improve the understanding, diagnosis, and treatment of EoE,” says Rothenberg. “The computers are now working on this project, around the clock, even when the researchers are sleeping, and are already uncovering new breakthroughs about the disease.”
NEXT STEPS
The research team plans to continue to feed more information into the computer, which is expected to improve the accuracy and precision of the diagnosis. The team also plans to harness the computer to learn more about the processes and causes of the disease.
The findings may also provide a universally applicable platform for diagnosing the disease and be readily available around the world, including locations where there is limited knowledge and experience with EoE.
ABOUT THIS STUDY
This work was supported by a grant from the American Federation for Aging Research, Israel Science Foundation (#1619/20); a grant from the National Institutes of Health (R01AI045898-21); the Campaign Urging Research for Eosinophilic Diseases (CURED) Foundation; and the Dave and Denise Bunning Sunshine Foundation.
Read more about the Cincinnati Children’s-Technion collaboration
Original title: | Machine Learning Approach for Biopsy-Based Identification of Eosinophilic Esophagitis Reveals Importance of Global features |
Published in: | Institute of Electrical and Electronics Engineers (IEEE) Open Journal of Engineering in Medicine and Biology |
Publish date: | June 16, 2021 |
Research By
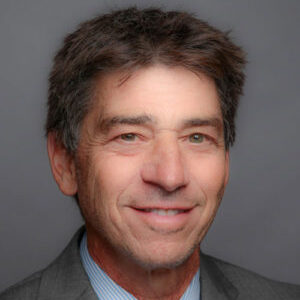
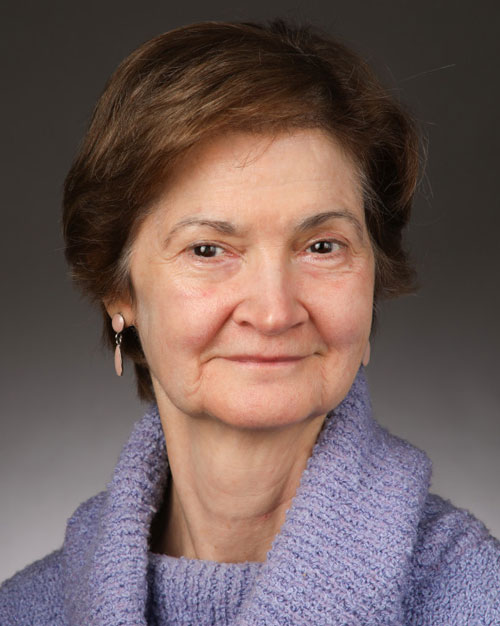