Cell Harmony: Computational Tool to Discover Origins of Pediatric Diseases
Research By: Nathan Salomonis, PhD | H. Leighton (Lee) Grimes, PhD
Post Date: September 17, 2019 | Publish Date: Sept. 16, 2019
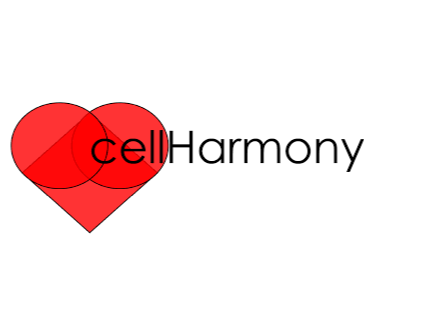
What are the differences between diseased and healthy cells, and what can they tell us about pediatric diseases? Researchers at Cincinnati Children’s have developed a new computational tool to automatically analyze and interpret cellular data, leading to a better understanding of pediatric diseases and potential therapies.
The new tool, cellHarmony, is described in the journal Nucleic Acids Research. cellHarmony was developed by researchers in the Salomonis Laboratory in the Division of Biomedical Informatics, in collaboration with co-corresponding author H. Leighton (Lee) Grimes, PhD, and scientists in the Bioinformatics Collaborative Services group.
Creating a “dating service” for cells
In recent years, single-cell sequencing techniques have enabled researchers to identify and compare cell populations. These techniques produce massive datasets, leading to a new challenge—analyzing and interpreting the data. Identifying discrete differences between diseased and healthy cells requires significant computational protocols and expertise.
The research team, led by Nathan Salomonis, PhD, designed cellHarmony to address this challenge. Using a community clustering and alignment strategy, cellHarmony matches single-cell transcriptomes to automatically identify differences in gene expression of cell populations.
The tool works by projecting one scRNA-seq dataset onto another, matching each cell in similar sample communities. Transcriptional differences highlight impacted gene pathways, giving researchers a better understanding of how genetic, chemical, and disease perturbations impact the body on a systems level.
“For each individual cell among tens of thousands of diseased cells from a single-cell RNA-Seq experiment, cellHarmony works to find its perfect match in a healthy reference dataset before uncovering essential differences,” says Salomonis. “We describe this as a ‘dating service’ for cells. At first, this approach attempts to match cells between datasets, but in the end, it exploits hidden differences that make the healthy and diseased cells distinct.”
Why do these differences in cells remain hidden to begin with? Since dozens of cell types may be present in one dataset, they can be challenging to compare.
“A single cell dataset may contain hundreds, thousands, or hundreds of thousands of cells, making the need to efficiently match cells between datasets computationally slow,” says Salomonis. “We found a work-around to this problem by finding cliques of cells in the compared datasets and matching the cliques before matching individual cells.”
In addition to making the alignment of datasets more efficient, cellHarmony automates all of the downstream comparison steps. This allows both experienced bioinformaticians and non-computational biologists to use the tool without additional expertise.
Uncovering novel disease mechanisms
To demonstrate that cellHarmony can discover known and novel cell-population impacts, researchers applied the tool in datasets from two diseases: myocardial infarction and Acute Myeloid Leukemia.
Myocardial infarction (MI)—commonly known as a heart attack—is the leading cause of death in developed countries. Decades of transcriptomic analyses have shown the impact of MI on cardiac tissue, but little is known about the impact on individual cells. Working with researchers in the Heart Institute, the team applied cellHarmony to a mouse model of MI, discovering novel disease regulatory gene networks that were active in surprising cell types. This gives insight on the cell populations in MI that are involved in therapeutic responses and causes of disease.
Acute Myeloid Leukemia (AML) is a type of cancer in which the bone marrow makes abnormal myeloblasts, a type of white blood cell. Researchers aimed to learn more about the diversity of cancer cell origins and response to therapy. In collaboration with the Division of Immunobiology, the team applied cellHarmony to mouse models and patient samples of AML. Resulting data identified potential disease biomarkers and pathways activated in the cells during chemotherapy.
“The hope is for such an approach to enable the discovery of novel disease mechanisms in poorly understood pediatric diseases,” Salomonis says, “which this tool is ultimately designed for.”
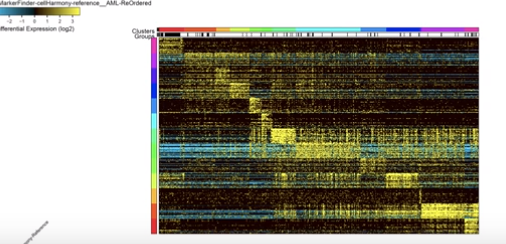
Original title: | cellHarmony: cell-level matching and holistic comparison of single-cell transcriptomes |
Published in: | Nucleic Acids Research |
Publish date: | Sept. 16, 2019 |
Research By
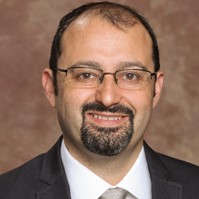
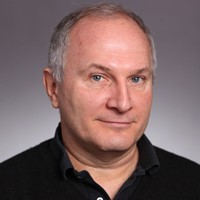