Predictive Model Maps Early-Stage Pandemic Infection Spread
Research By: Shelley Ehrlich, MD, ScD, MPH
Post Date: April 19, 2021 | Publish Date: March 8, 2021
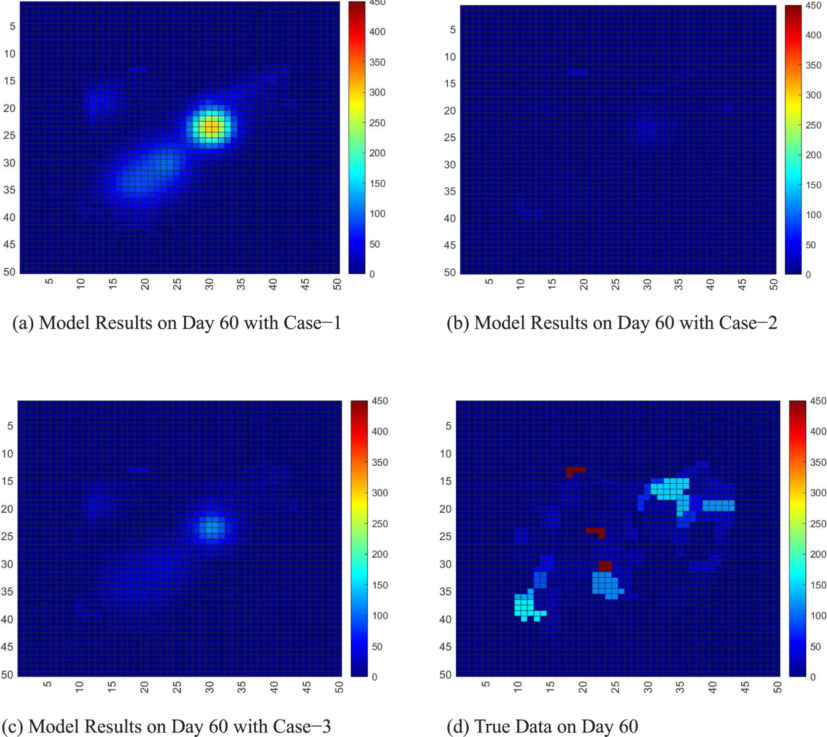
The time lag that occurs between the beginning of a pandemic and the availability of vaccines and therapeutics takes an inevitable toll in terms of economic costs and human lives lost. The extent of those losses can be mitigated by non-pharmaceutical interventions (NPIs) such as social distancing, isolation, face masks and hand washing.
In the COVID-19 pandemic, NPIs demonstrated effectiveness–when employed–in slowing the spread of the virus. However, policy makers at local, regional and national levels also faced significant challenges in implementing NPIs.
Now, researchers are developing advanced mathematical models that factor in the real-world lessons from the COVID-19 pandemic to help us better prepare for future pandemics.
Researchers from Cincinnati Children’s, the University of Cincinnati, and the University of Minnesota Duluth detailed their updated modeling methods March 8, 2021, in the journal ISA Transactions.
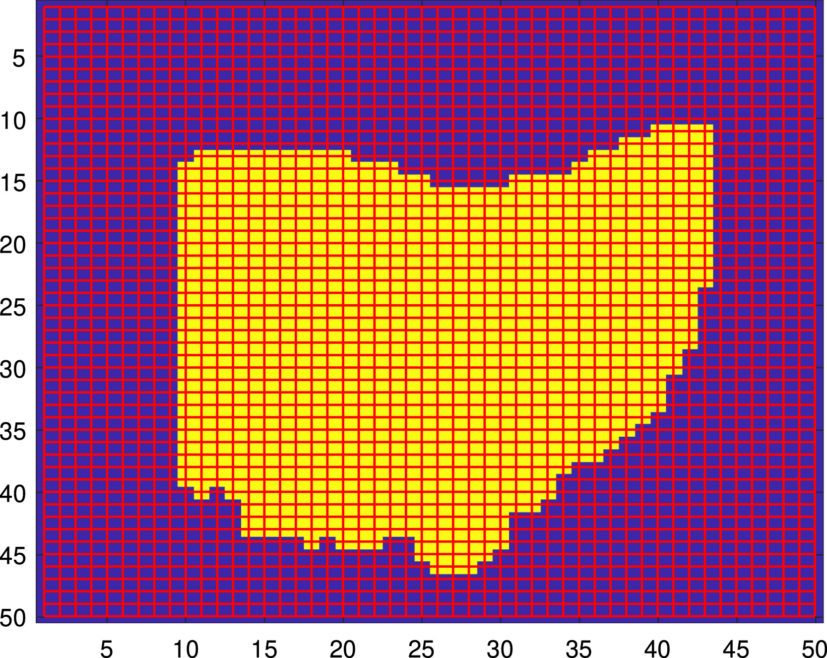
Understanding Infection Spread
“The role of uncertainties that drive infection spread, while significant, have yet to be well understood,” says corresponding author Shelley Ehrlich, MD, ScD, MPH, of the Division of Biostatistics and Epidemiology at Cincinnati Children’s. “Our approach uses novel mathematical and computational frameworks to create a better understanding of how uncertainties—such as human behavior, mobility, transmissibility of the pathogen, unexpected variants, and individual susceptibility—affect an infection spread.”
First, the team developed a partial differential equation-based Susceptible–Latent–Infected–Recovered (SLIR) model for the dynamics and control of a pandemic. Next, they validated the model using empirical COVID-19 data within a period of 45 days from the state of Ohio. Infection data from the first 30 days provided a basis to optimize the model parameters. For the next 15 days, researchers found that the model was able to accurately predict the data.
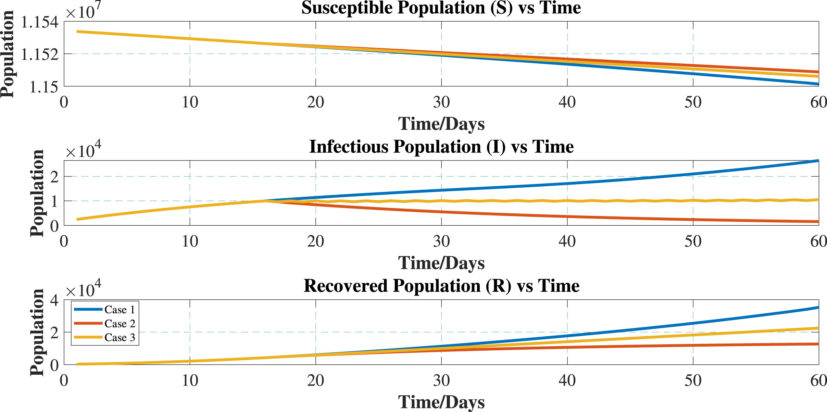
To investigate the efficacy of NPIs, the team then introduced control inputs representing social distancing and mask mandates. Results showed that these controls had a significant effect in mitigating the viral spread.
Guiding Policy Decisions
Since the model was validated using real-world data, it can be a useful tool to forecast the spread of disease. The control parameters not only signify the importance of NPIs, but also provide an effective baseline to analyze their effects based on timing, location, and levels.
“Improved predictive models can be very helpful in shaping interventional public health policy in the face of scarce data,” says Ehrlich. “This could help policy makers at the local, state, and federal levels quickly make the appropriate public health recommendations and also know when to loosen them.”
*As vaccines become more widely available for COVID-19, prioritized distribution remains an important issue. Predictive models can be helpful in developing vaccination strategies by improving our understanding of the spatiotemporal dynamics of pandemics—both as the current pandemic changes over time and as future pandemics emerge.
What’s Next?
With this predictive model as a baseline, the research team sees potential for more sophisticated predictions by utilizing larger datasets and adding functionalities.
“Our latest results suggest that the model works even better with Hamilton County data thanks to our collaboration with the Hamilton County Health Commissioner,” says Ehrlich. “In ongoing further research, we seek to deeper investigate the analytical aspects, characterization of super-spreader events, appearance of new variants, and the impact of vaccinations.”
About This Study
Co-authors of this study include Manish Kumar, PhD (University of Cincinnati Department of Mechanical and Materials Engineering, College of Engineering and Applied Science), Subramanian Ramakrishnan, PhD (University of Minnesota Duluth Department of Mechanical and Industrial Engineering) and their students, Faray Majid, Michael Gray, and Aditya Deshpande.
Research By:
Division of Biostatistics and Epidemiology
Research interests include environmental molecular and perinatal epidemiology; obstetrics, gynecology and reproductive medicine; endocrine disrupting chemicals; epigenetics; novel biomarkers; fetal programming of childhood diseases.
Original title: | Non-Pharmaceutical Interventions as Controls to mitigate the spread of epidemics: An analysis using a spatiotemporal PDE model and COVID-19 data |
Published in: | ISA Transactions |
Publish date: | March 8, 2021 |
Research By
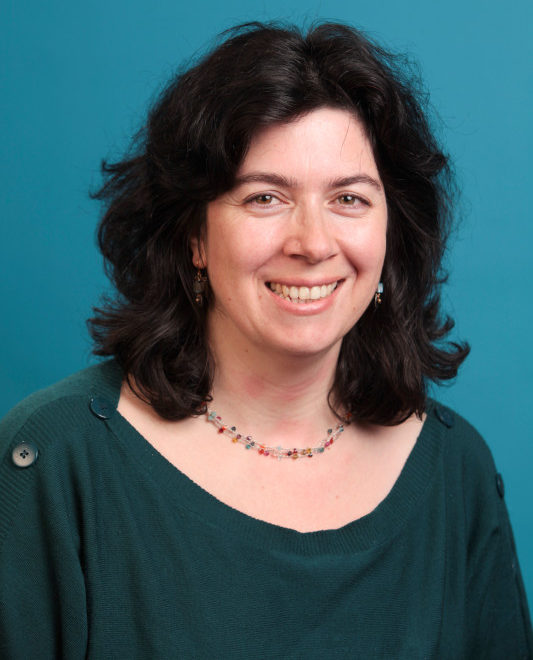