New Tool Provides Low-Cost, High-Quality Means to Map Transcription Factor Binding Genome-Wide
Research By: Emily Miraldi, PhD
Post Date: April 5, 2023 | Publish Date: Jan. 31, 2023
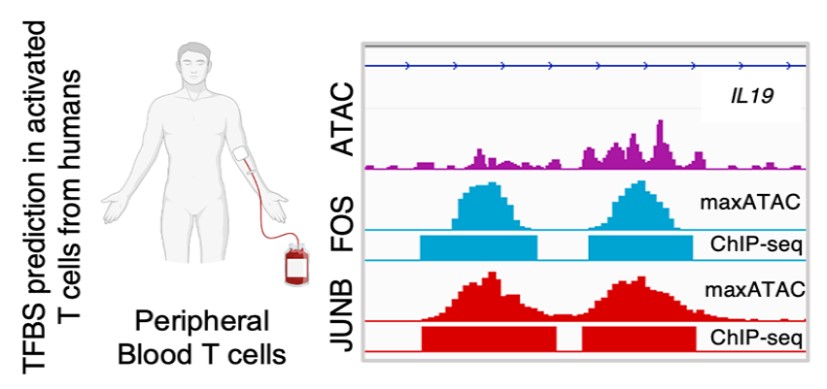
Scientists at Cincinnati Children’s develop maxATAC, a computational modeling tool that can be applied to understand disease mechanisms across cell types
Transcription factors (TF) are proteins that regulate gene expression based on precisely where they bind to chromatin, strands of DNA decorated with proteins and other molecules that house our genetic code within the nucleus of every human cell.
So far, scientists have identified more than 1,600 human transcription factors, which orchestrate regulatory networks that tune gene expression in response to key signals of growth, development, and disease response.
Two recent technological advances have allowed researchers to learn much more about the roles TFs play in health and disease. ChIP-Seq data measure where along the genome a specific TF is bound. Meanwhile, ATAC-seq data correlates with TF binding, revealing “accessible” chromatin regions, maintained open by TFs and accessible for the binding of additional TFs.
While, in theory, it would be better to directly measure binding of all TFs with ChIP-seq, in practice, this is not feasible. Experimentally measuring just one TF requires obtaining millions of cells of the targeted cell type, and the experiment often requires weeks of lab work to optimize the results. Making matters worse: There are hundreds of TFs expressed in a given cell type and their binding sites often change, depending on the context (infection, aging, etc.). Thus, hundreds of ChIP-seq experiments are needed to characterize even a single regulatory landscape.
For rare cell types and clinical samples from disease contexts, hundreds of millions of cells often cannot be obtained.
In contrast, the correlative ATAC-seq data is feasible from as few as hundreds of cells, from population and single-cell experiments. From only a single experiment, the binding of hundreds of TFs can be inferred computationally.
However, the quality of these predictions is low relative to experimental measurement by ChIP-seq. Thus, while the savings are huge (about $300 for TF binding prediction from ATAC-seq vs. about $50,000 for 100 ChIP-seq experiments), TF binding prediction from ATAC-seq is limited due to a lack of high-quality computational models.
A faster, less costly, more accurate alternative
To address such obstacles, Emily Miraldi, PhD, and colleagues at Cincinnati Children’s created a suite of computational models, dubbed maxATAC, that use cutting-edge deep learning to predict transcription factor binding sites from ATAC-seq. These deep learning models, available for 127 TFs, were carefully built and validated by the Cincinnati Children’s team.
“With maxATAC, it becomes possible for researchers to explore TF binding for a hundred TFs in any human cell type with ATAC-seq data. This is feasible even from single-cell ATAC-seq data, dramatically decreasing costs and starting material requirements,” Miraldi says.
The team demonstrated maxATAC’s capabilities, to generate “in silico” ChIP-seq for immune cells derived from patients with atopic dermatitis, a chronic disease that causes inflammation, redness and irritation of the skin. maxATAC was used to predict binding sites for 105 TFs, in a context where experimental measurement of hundreds of TFs was not feasible. This analysis predicted potential disease mechanisms, as several allele-dependent TF binding sites were identified at genetic risk loci for atopic dermatitis.
The new tool has the potential to improve our understanding of disease mechanisms and gene regulation in disease and physiological contexts, Miraldi says.
Next Steps
With models available for 127 human TFs, this is the largest collection of advanced TF binding models for ATAC-seq, and the research team is working to add more models in the years to come.
The maxATAC models will aid in the elucidation of gene regulatory networks, which, long term, could aid in identifying new drug targets for a wide range of diseases as well as study how to refine existing medication regimens to improve effectiveness and/or reduce adverse events.
Miraldi and colleagues have decided to publish this user-friendly tool as an open resource for any researcher who has access to ATAC-seq data. See article in PLOS Computational Biology.
Original title: | maxATAC: Genome-scale transcription-factor binding prediction from ATAC-seq with deep neural networks |
Published in: | PLOS Computational Biology |
Publish date: | Jan. 31, 2023 |
Research By
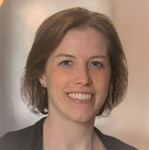
The Miraldi lab’s focus is mathematical modeling of the immune system from high-dimensional genomics measurements. In close collaboration with experimental immunologists, we seek to learn how diverse immune cells sense and respond to their environment in both health and disease.