Informatics Technologies Uncover Pediatric Substance Use Information
Research By: Yizhao Ni, PhD | Sarah Beal, PhD
Post Date: August 2, 2021 | Publish Date: August 1, 2021
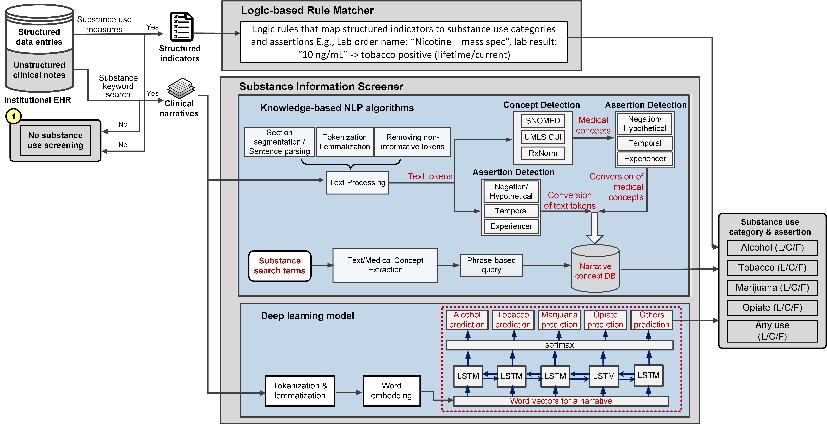
“There is a critical need for developing an efficient and accurate approach to detect substance use information from electronic health records (EHRs)…”
–Yizhao Ni, PhD
During interactions with healthcare providers, adolescents are often screened for substance use—including alcohol, tobacco, marijuana, and opiates. Are they currently using? Have they used in the past? Do they have a family history of use?
Over time, the importance of this information continues to grow, but it can be difficult to uncover from clinical notes. Is there a better way to find and track the data?
Researchers at Cincinnati Children’s are harnessing natural language processing (NLP) and deep learning technologies to develop a new approach for identifying substance use information. The system, Automated Substance Use Detection System (ASUDS), is described in a study published August 1, 2021, in the Journal of the American Medical Informatics Association (JAMIA).
Why Adolescent Screening Matters
Substance use can start early, with initiation occurring in adolescence and increasing into young adulthood. Pediatric healthcare providers play a pivotal role in identifying initiation of substance use, monitoring use over time, and providing referrals to treatment when necessary.
However, the questions providers use for screening are generally unstandardized, meaning that descriptions of substance use vary widely. This information is then documented in unstructured clinical notes, where the process of retrieval is complex and time-consuming.
“Efforts to standardize screening and documentation are slow, and implementation continues to be a challenge,” says corresponding author Yizhao Ni, PhD, of the Division of Biomedical Informatics at Cincinnati Children’s. “There is a critical need for developing an efficient and accurate approach to detect substance use information from electronic health records (EHRs) in order to provide evidence-based prevention and intervention strategies to reduce substance use in our community, and to study change in substance use over time.”
While previous studies have presented NLP algorithms for substance use screening, all have focused on adult patients. Researchers at Cincinnati Children’s recognized the need for algorithms catered to the pediatric setting, where data is often sparse, diagnostic and billing codes are unreliable, screening characteristics like family history are especially important—and the opportunity to prevent substance use is greatest.
A Smarter Way to Screen
The research team began by rethinking how to best support pediatric substance use screening. They decided to meet providers where they were—instead of relying solely on structured data, they would also improve access to the unstructured clinical notes many providers already collect.
As part of a larger study to understand the emergence of substance use among adolescents in foster care, researchers collected data on 3,890 participants aged 10-20 years who had at least one substance use screening. Data came from both EHRs and clinical notes created during encounters.
First, researchers manually annotated substance use information from clinical notes. Two data analysts reviewed each narrative to identify five categories of substance use—alcohol, tobacco, marijuana, opiate, and any use. If these substances were mentioned, the analysts further classified the information into three assertions—lifetime, current, or family use. By merging this unstructured data with structured data from EHRs, researchers created a “gold-standard set” to evaluate their automated approach.
This approach—the ASUDS—utilizes a logic-based rule matcher to classify data from EHRs into screening results. Next, an NLP and deep learning-based substance information screener detects categories and assertions from clinical notes. When the results merge, they create a final prediction of whether substance use screening occurred and the associated results for each encounter.
After comparing ASUDS with the gold-standard set, researchers found that the system achieved high detection capacity. These results represent the first step toward developing an accurate and scalable informatics-based solution to support substance use screening.
Opportunities to Reduce Risk
In the clinical setting, this system could provide a more complete picture of substance use history for each patient—whether they have been screened, if they are engaging in substance use, and how their use is changing throughout adolescence.
“Given its high performance in this stage of development, ASUDS holds great potential to facilitate research and healthcare delivery addressing substance use screening in adolescence,” says coauthor Sarah Beal, PhD, scientific director of child welfare research at the CHECK (Comprehensive Health Evaluations for Cincinnati’s Kids) Foster Care Center at Cincinnati Children’s. “Ultimately, when combined with prevention and intervention, the system could reduce risk of substance use disorders across the lifespan.”
Next, researchers aim to validate the system in diverse patient populations to understand and improve its generalizability. The team will focus on evaluating the system’s effect in mitigating screening bias to avoid perpetuating racism and inequity in healthcare. Once reliability and generalizability are established, the system can be transferred to a production environment.
“When we are able to successfully share screening information with clinicians, they will be equipped to ensure that all patients are asked about substance use and offered evidence-based interventions,” says Beal. “Given the significant negative impact of substance use disorders in our community, opportunities to prevent and reduce substance use during this critical period in development are exciting and promising.”
About the Study
Co-authors for this study also included Alycia Bachtel, BA, and Katie Nause, BS, of Cincinnati Children’s.
This work was supported by the National Institutes of Health [grant numbers: 1K01DA041620, 2UL1TR001425-05A1; 1R01HD103630], and the Patient-Centered Outcomes Research Institute [grant numbers: PCORI/PCS-2018C1-11111]. YN was also supported by internal funds from Cincinnati Children’s Hospital Medical Center.
Original title: | Automated detection of substance use information from electronic health records for a pediatric population |
Published in: | Journal of the American Medical Informatics Association |
Publish date: | August 1, 2021 |
Research By
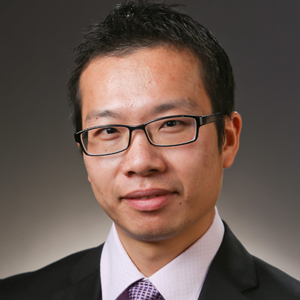
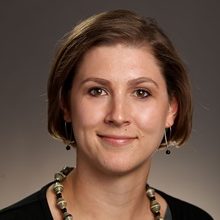