Artificial Intelligence Improves Ability to Use MRI to Diagnose ADHD
Research By: Lili He, PhD
Post Date: December 31, 2019 | Publish Date: Dec. 11, 2019
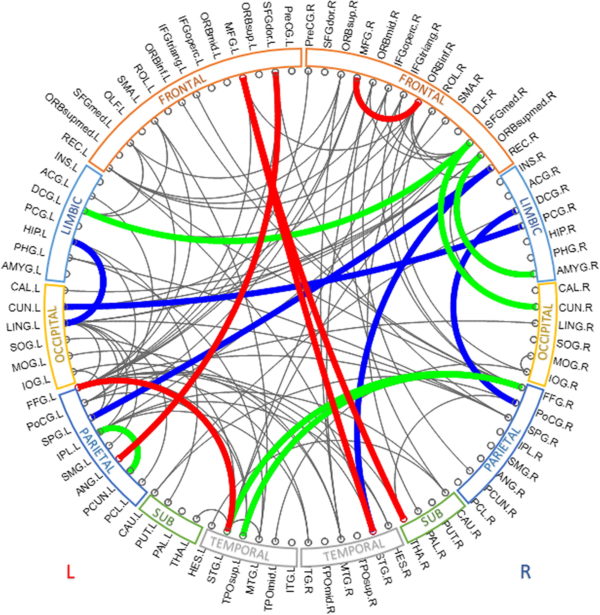
“Our results emphasize the predictive power of the brain connectome.”
—Lili He, PhD
Deep learning, a type of artificial intelligence, can boost the power of MRI in predicting attention deficit hyperactivity disorder (ADHD), according to a study led by Cincinnati Children’s scientist Lili He, PhD, and published in Radiology: Artificial Intelligence.
The findings are based on advanced functional MRI techniques that have allowed scientists to create maps of brain activity networks called connectomes. Experts are working to make these maps accurate enough to pinpoint how ADHD disrupts connections between brain regions.
He and colleagues at Cincinnati Children’s and the University of Cincinnati College of Medicine pushed this effort forward by using artificial intelligence to help analyze multiple connectomes at multiple scales. The result: a significantly more sophisticated model of ADHD brain activity.
“Our results emphasize the predictive power of the brain connectome,” He said in a media release from the journal. “The constructed brain functional connectome that spans multiple scales provides supplementary information for the depicting of networks across the entire brain.”
The data used to build and test the model came from a global competition called the NeuroBureau ADHD-200 project, which was held in 2011 to accelerate the hunt for ADHD biomarkers.
Read the announcement from Radiology: Artificial Intelligence
Read coverage at Futurism.com
Read coverage at RadiologyBusiness.com
Original title: | A Multichannel Deep Neural Network Model Analyzing Multiscale Functional Brain Connectome Data for Attention Deficit Hyperactivity Disorder Detection |
Published in: | Radiology: Artificial Intelligence |
Publish date: | Dec. 11, 2019 |
Research By
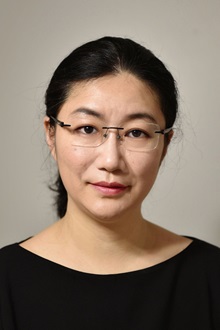